The Green Sheet Online Edition
July 7, 2025 • 25:07:01
Insider's report on payments
The mainstreaming of cryptocurrencies
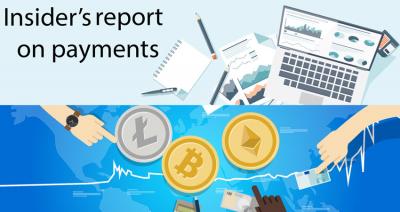
It began like a fad, or perhaps a new way to bypass the prying eyes of government, but now cryptocurrency is taking off like a rocket ship. The price of a single bitcoin, which in 2010 was about a dime, these days well exceeds $100,000. In the interim years, however, the value has ballooned and deflated numerous times.
Recently, I had a conversation with Keith Riddle, general manager at Payfinia Inc., a technology platform created to help community financial institutions take advantage of embedded payments and digital wallets, about the trend. He agreed with me that they won't replace credit and debit cards, at least not initially.
Stablecoins were created to address the wild gyrations witnessed in the crypto market. This is done by pegging the value of a crypto asset to a more stable asset, such as fiat currencies like the U.S. dollar.
The first big hint I had that crypto had legs was when headlines were made about a Trump Administration move to allow crypto holdings to serve as a federal mortgage asset. "After significant studying, and in keeping with President Trump's vision to make the United States the crypto capital of the world, Today I ordered the Great Fannie Mae and Freddie Mac to prepare their businesses to count cryptocurrency as an asset for a mortgage," U.S. Federal Housing Director William Pulte posted to X on June 25, 2025.
Fannie Mae (the Federal National Mortgage Association) is a government-sponsored organization that purchases mortgage loans from lenders, thereby freeing up lenders to make more mortgage loans. Freddie Mac (the Federal Home Loan Mortgage Corporation), serves a similar purpose, packaging the mortgages it purchases into mortgage-backed securities for sale to investors.
Historically, cryptocurrencies were excluded from underwriting criteria for any number of reasons, including volatility, the inability to easily verify assets and regulatory uncertainty.
Today, numerous regulatory agencies have jurisdiction over cryptocurrencies. The Securities and Exchange Commission, for example, regulates those it considers investment contracts.
The Commodity Futures Trading Commission regulates those that are considered commodities, like bitcoin. Even some states have a say. New York businesses involved with cryptocurrencies, for example, require special state-issued licenses.
It's not just a domestic issue either. The Financial Action Task Force, which leads global action against money laundering and terrorist financing warned on June 26 that some countries aren't doing enough to keep bad actors from financing criminal activities with cryptocurrencies.
"With virtual assets inherently borderless, regulatory failures in one jurisdiction can have global consequences," the FATF noted.
Stablecoins: overcoming negativity
The FATF stated that mass adoption of stablecoins by bad actors is particularly concerning.
Stablecoins are cryptocurrencies tied to stable assets (like the U.S. dollar) that are held in blockchain ledgers separate from traditional financial institution accounts.
On Feb. 21, 2025, bad actors associated with the Democratic People's Republic of Korea (North Korea) used social engineering techniques and malicious coding to access wallet infrastructures to steal $1.46 billion from the cryptocurrency exchange ByBit. The FATF also found a "significant uptick" in the use of virtual assets, like stablecoins, to perpetrate frauds and scams, with one estimate putting the total lost in 2024 at $51 billion.
The Bank for International Settlements, the central bank of central banks, offered its own dire assessment. It wrote in a June 2025 paper issued that stablecoins "perform poorly" especially in terms of becoming a "mainstay" of the monetary system. And the potential for criminal activity is only one of the reasons.
There's the lack of issuer oversight, and the need for a profitable business model that involves liquidity or credit risk. "If stablecoins continue to grow, they could pose financial stability risks," BIS asserted.
The independent Community Bankers Association of America pointed to the BIS assessment in a June 25 news release, raising concerns about disintermediation of community banks if stablecoins take off.
It urged lawmakers, who are currently considering legislation that would erect regulatory guardrails around stablecoins, to make sure that any guardrails adopted do not lead to negative economic consequences for its members.
Stablecoins transformative
The propensity for problems aside, stablecoins are becoming increasingly mainstream.
Zach Abrams, co-founder of stablecoin platform Bridge, had this to say following the company's acquisition by the payments platform Stripe: "Stablecoins aren't the future – they're already transforming how people move money today."
In a May 20 interview broadcast by CNBC, Abrams equated the attraction to and growth of stablecoins to the 20th century rise of credit cards. "We think stablecoins are an entirely new money movement platform," he said.
But stablecoins represent a lot more value than credit cards did at this stage of their development. Abrams estimated the current market capitalization of stablecoins to be $400 billion.
"That could easily grow to $2 trillion," he said, noting that as of July, the market capitalization of Visa and Mastercard, combined, was $1.22 trillion. "The market has surprised us in terms of how quickly stablecoin adoption has come around, especially over the last six months," he added.
Stablecoins and the financial mainstream
Stripe isn't the only financial services company interested in integrating stablecoins with traditional financial instruments. Visa is teaming up with Bridge on a new card-issuing product. Fintech developers can now offer stablecoin-linked Visa cards to their end customers in multiple countries through a single API integration. Cardholders will be able to make everyday purchases from stablecoin balances at any merchant location that accepts Visa cards.
"We're focused on integrating stablecoins into Visa's existing network and products in a frictionless and secure way," said Jack Forestell, Visa chief product officer.
The integration enables issuance of new card programs in multiple countries at once, starting with Argentina, Columbia, Ecuador, Mexico, Peru and Chile. The focus on Latin America aims to address the growing demand there among consumers and businesses to utilize stablecoins to store value and fund everyday purchases. Plans are to expand coverage to Europe, Africa and Asia in coming months.
In an April 28 statement, Mastercard said it was "advancing the future of payments" by allowing consumers and businesses to use stablecoins "as easily as the money in their bank accounts." It announced a collaboration with crypto wallet OKX to launch the OKX card.
The card company also is teaming up with payment company Nuvei and the crypto platform Circle to give merchants the option to receive payouts in the form of stablecoins, such as Circle's USDC, regardless of how consumers choose to pay. Mastercard also is working with blockchain fintech Paxos to enable this functionality across Paxos-issued stablecoins.
Even lesser known companies, like Bolt, a checkout, identity and payments platform, are getting into the act. Bolt, on June 27, touted stablecoins as a benefit to both sides of payment transactions. "For merchants, it means faster settlement, lower transaction fees, and easier cross-border payouts—especially relevant for marketplaces," the company wrote.
"For shoppers, stablecoins offer a private, instant and borderless way to pay, no credit card, bank account or international fees required." "When it comes to blockchain and digital assets, the benefits for mainstream use cases are clear," said John Lambert, chief product officer at Mastercard.
"To realize this potential we need to make it easy for merchants to receive stablecoin payments and for consumers to use them."
Still, stablecoin payments won't replace credit card transactions, at least not any time soon. Riddle noted that while instant payments and same-day ACH have grown substantially in recent years, they have not been displacing existing payment methods. "I think we're going to see shifting preferences among consumers and businesses to leverage a payment stream that is applicable to their use case," he said.
Hence the interest in stablecoins as expressed by large retailers, like Walmart and Amazon. "The combination of stablecoins and instant payments certainly will have an impact on credit and debit card interchange for issuers, over time," Riddle said. The emphasis on "over time."
Riddle pointed out that the vast majority of stablecoin transactions thus far have been for business-to-business payments, particularly for cross-border transactions. That's because stablecoins streamline foreign exchange processes by providing a standard blockchain framework for both buyers and sellers.
Riddle added that crypto platforms like Coinbase and Robinhood already connect to instant payment networks, allowing businesses and consumers to quickly move money in and out of their digital wallets. So clearly, stablecoins have feet, and will be taking off fast once the GENIUS Act is passed.
Stablecoin competition
There are bank alternatives to traditional stablecoins. JPMorgan Chase has JPM Coin. It also introduced a U.S. dollar deposit token, JPMD, for its institutional clients. The idea is to allow clients to easily transfer money across borders past the close of markets. It operates on a blockchain platform, and the tokens are held by actual deposits held by the bank.
Other major banks, like Bank of America, Citigroup and Wells Fargo, are rumored to be in negotiations about a shared stablecoin. This may be driven, at least in part, by perceptions that interchange revenues will eventually decline as stablecoins take hold.
Riddle predicted that community banks will follow suit, because their interchange revenues will be impacted, and they will want to offer opportunities for lower-cost value exchanges for their merchants.
Patti Murphy is senior editor at Green Sheet and president of ProScribes Ink, (www.proscribes.net). You can also follow her blog, Today in Payments, at (Todayinpayments.com), and her podcast, Merchant Sales Podcast, co-hosted with James Shepherd at www.ccsalespro.com/podcast.
Notice to readers: These are archived articles. Contact information, links and other details may be out of date. We regret any inconvenience.